New Solutions
AI Data Lake: Breaking Silos and Accelerating Intelligence
Huawei has launched a three-layer AI data lake solution to break down the data silos that are preventing many enterprises from reaping the benefits of AI models.

By Michael Fan, Director, Data Storage Marketing Execution Dept, Huawei
Since the release of ChatGPT in November 2022, large AI model technology has been on a rapid upward trajectory, with model training emerging as the driving force behind technological progress. However, the data volume and diversity of data types involved in model training are increasing exponentially, meaning that data silos pose a major barrier to progress.
Many organizations are struggling to see the way forward.
Huawei's AI data lake solution, however, does not just create bridges between data silos, it lights the way to a more intelligent world and a new era of AI innovation and development.
Trends and challenges of large AI model services
In 2023, Google released the Gemini multimodal model, which is able to understand, operate, and integrate different types of information, including text, code, audio, images, and video. In February 2024, OpenAI released a video model called Sora, which can create realistic scenes from text instructions. By combining a diffusion model with the large language model, Sora exhibits amazing 3D consistency when learning from the physical world.
The speed with which large AI models have developed has far exceeded people's expectations. Witnessing the progression from ChatGPT to Gemini and now to Sora, we have identified two key trends:
- Trend 1: The shift from pure NLP models to multimodal models has brought a corresponding shift in training data. Training datasets now encompass a mix of text, images, audio, and video, rather than text alone. This means that the volume of data required to train today's most advanced models is a staggering 10,000 times greater than was required for earlier models (as shown in Figure 1).
- Trend 2: Computing power, algorithms, and data are the three core elements of large AI models. Through the stacking of computing power, aggregation of data, and parameter scaling (from hundreds of billions to trillions, and even up to 10 trillion), complex behaviors emerge within the framework of deep learning algorithms. For example, in a video released by Sora, we witness a stylish woman strolling down a street. As she moves, the perspective on the street scenes behind her seamlessly adjusts. As she walks by, objects and other pedestrians are momentarily obscured, and then reemerge, maintaining impeccable 3D consistency and object permanence, faithfully replicating how the world is perceived through human eyes.
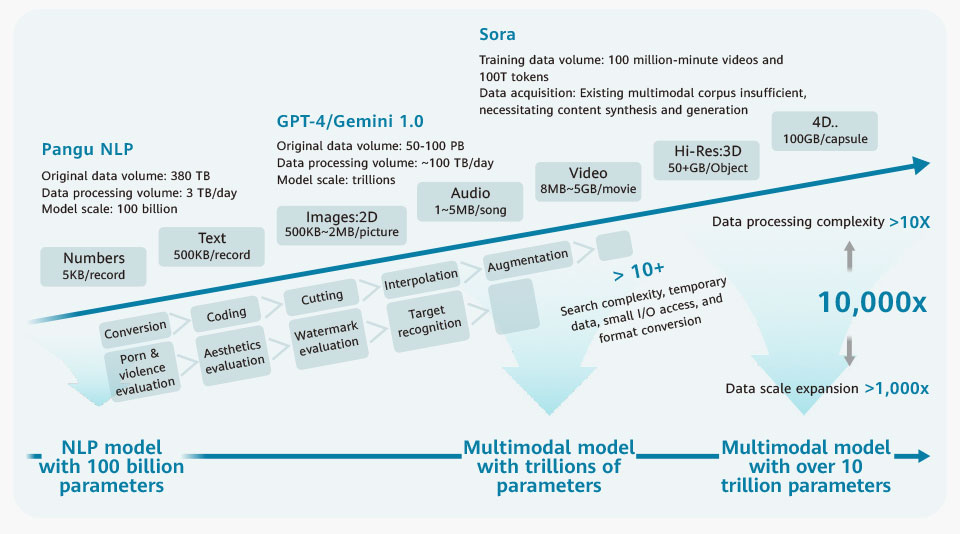
Figure 1: Multimodal models for exponential growth in the volume of training data
The transition from unimodal to multimodal models will create a deluge of data, and a solution is urgently required that can integrate scattered data resources. As a way of presenting the real world, data serves as the cornerstone of training large AI models. With deep learning algorithms, the quantity and quality of data determines how far AI training will go. However, most data owners prioritize the efficient access of data by service applications, often overlooking where data is stored. Similarly, most data administrators focus on whether data is effectively stored, and may neglect data ownership and types. As a result, data ends up siloed in different data centers. One carrier we spoke to has seen the total amount of data they need to store soar to hundreds of PBs in recent years, and hundreds of TBs of new data is generated each day. However, this data is scattered across multiple data centers.
To provide as much data as possible for model training, the carrier's technical department has to migrate or replicate the data from scattered data silos across regions. As a result, the data preparation process is prolonged, accounting for over 50% of the entire model training duration.
Therefore, the biggest challenges and primary considerations for constructing large AI model infrastructure revolve around eliminating data silos and effectively gathering data in one place, swiftly transforming collected datasets into model training materials, and ensuring that AI computing power has efficient access to training materials.
New requirements for data storage and management
The ideal AI data infrastructure should be able to support the key phases of large AI model training, such as data ingestion, data preprocessing, and model training, to provide high-quality data services. To achieve this goal, at least two layers of data infrastructure should be considered: the storage device layer and the data management layer.
Storage device layer
When dealing with a large amount of heterogeneous data from multiple sources, especially in multimodal AI training scenarios, the ideal storage device layer should feature multi-protocol interworking, high I/O performance, and easy scalability to address various challenges and support the following pivotal phases of training large AI models:
- Data ingestion: In this phase, data is often scattered across different silos, stored in various formats, and accessed through different protocols. To efficiently centralize heterogeneous data from different sources, data storage devices need to support various data formats and access protocols, and deliver high write bandwidth. Additionally, storage solutions need to be both flexible and scalable, while also remaining cost-effective, in order to handle new data sources that may be incorporated into training at any time. While data formats and access protocols may vary during the data ingestion phase, servers only access data through the file interfaces during the training phase. Therefore, ideal storage hardware should support multi-protocol interworking to ensure that underlying data can be accessed by different protocols or interfaces, thereby eliminating the data replication caused by protocol conversion.
- Data preprocessing: Data preprocessinginvolves the cleansing, transformation, augmentation, and standardization of diverse data to yield high-quality training materials from massive volumes of raw data. In this phase, a large amount of temporary data is generated due to the diversity of preprocessing tools, resulting in data expansion. Therefore, storage devices should offer extensive shared storage capacity while also delivering high read/write bandwidth and supporting random access to expedite preprocessing.
- Large AI model training: In the model training phase, the performance of storage devices in tasks such as training data loading and checkpointing directly affects training efficiency. Although the volume of pre-processed training data is small, high file access performance (OPS and IOPS) and low latency are required to ensure quick data loading and avoid wasting GPU/NPU computing power. Saving checkpoints during the training process is critical to ensure that, in the event of an interruption, training can be resumed rather than started from scratch. Therefore, it requires high write bandwidth for fast and frequent archiving and more stable training.
Data management layer
Based on the flexible capacity expansion and high hybrid-load performance provided by the storage device layer, the data management layer further provides advanced data management capabilities for AI training. It helps data owners and administrators maximize data value in a more efficient manner from three dimensions: visibility, manageability, and availability.
- Visibility: Data asset owners and administrators should have a comprehensive understanding of their data, including data storage location, data volume, and data types, akin to having a data map. Considered from this dimension, owners and administrators can quickly identify which data needs to be collected based on this map.
- Manageability: After determining the data to be collected, a mechanism is required to implement policy-based data flow. For example, a policy may be used to define the source and target of data flow, start and end time window, maximum rate limitation, and minimum rate guarantee, facilitating the management of data.
- Availability: Raw data needs to be preprocessed and converted into training data. While there are numerous tools for data preprocessing, it is still important to have a data preprocessing framework that works in synergy with the storage device layer. Such a framework should help users streamline data preprocessing and enhance processing speed to improve data availability.
Core capabilities of AI data infrastructure
The ideal AI data infrastructure should have the core capabilities outlined in Figure 2.
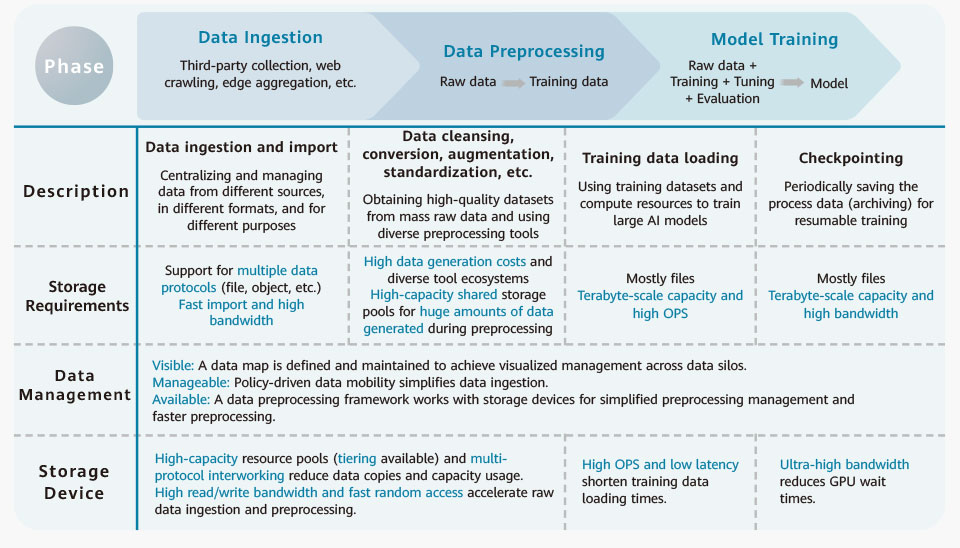
Figure 2: Core capabilities of AI data infrastructure
To sum up, three key features are indispensable:
-
High performance
High-performance data infrastructure underpins and accelerates every phase of large AI model training, from data ingestion and preprocessing in the early stage to training data loading and periodic checkpointing in the model training phase. High performance in this context is comprehensive and includes both high OPS and low latency for random access and high read/write bandwidth for sequential access. -
High capacity
The data ingestion and preprocessing phases involve the intensive reading, writing, and generation of temporary data, which creates storage challenges. Data infrastructure therefore needs to offer flexible and non-disruptive scale-out and tiering capabilities. This ensures a balance between cost and capacity, while delivering the necessary bandwidth, IOPS, and OPS to handle sequential and random data access patterns. -
Exceptional ease-of-use
Data infrastructure should feature global data management, efficient data mobility, AI platform and toolset collaboration, and optimization and enhancement tailored for large AI model training. This would accelerate AI training and learning by ensuring that data is visible, manageable, and available.
AI data lake solution
Huawei has been actively engaging in large AI model training with customers in diverse industries, including carriers. Over the years, we have gained extensive expertise in AI data infrastructure. Recently, we have launched an AI data lake solution to help customers solve the problems encountered in the deployment of data infrastructure for large AI model training. This enables our customers to focus on model development and training. The architecture of the Huawei AI data lake solution, as shown in Figure 3, consists of three layers: a data storage layer, a data fabric layer, and a data service layer.
Data storage layer
In this layer, data is stored in different data centers.
In each data center, data is intelligently stored in the hot and warm tiers. The hot tier is powered by the OceanStor A series, which is Huawei's high-performance storage specially designed for large AI model training. It can scale out to thousands of nodes. The warm tier uses Huawei's OceanStor Pacific scale-out storage to handle mass unstructured data. Intelligent tiering can be implemented between the OceanStor A series and the OceanStor Pacific series. That is, in the same storage cluster, the OceanStor A series nodes form a high-performance storage tier, and the OceanStor Pacific series nodes form a high-capacity storage tier. The two tiers are combined to externally present a unified file system or object bucket that supports multi-protocol interworking (one copy of data can be accessed through multiple protocols). Internally, data is intelligently and automatically tiered to achieve an optimal balance between capacity, performance, and cost.
A data replication relationship can be created between storage clusters across data centers to facilitate reliable and on-demand data mobility between data centers. This ensures the data device layer is fully prepared to support data ingestion for large AI model training.
Data fabric layer
The data fabric layer creates a seamlessly interconnected network to facilitate data mobility. It makes data visible, manageable, and available, helping large AI model training derive value from data.
Huawei uses a software layer powered by Omni-Dataverse to achieve data visibility, manageability, and availability. Omni-Dataverse is an important component of Huawei's Data Management Engine (DME). It provides the unified management of metadata on Huawei storage devices across different data centers to form a global data asset view. Omni-Dataverse also invokes interfaces on storage devices to control data movement. Omni-Dataverse executes actions based on user-defined policies, and can implement GPU/NPU-storage passthrough and intelligent file prefetching on demand to ensure training data is readily available, minimizing computing power wait times.
This makes data ingestion and model training much more efficient, improving cluster utilization.
Data service layer
The Huawei AI data lake solution provides commonly used service frameworks at the data service layer, including data preprocessing, model development, and application development frameworks.
The data preprocessing framework performs data cleansing, conversion, augmentation, standardization, and other preprocessing operations. Large AI model customers can integrate their algorithms and functions into this framework for simplified preprocessing management. Furthermore, customers can choose an alternative framework.
Similar to data preprocessing, model development and application development are the other two frameworks provided by Huawei for customers' convenience, which they can select on demand.
The Huawei AI data lake solution is built on years of experience and expertise in large AI model training. It helps enterprises eliminate data silos, achieve smooth data mobility, and implement a data fabric between data applications and storage devices, making data visible, manageable, and available. As large AI models continue to evolve from unimodal to multimodal, the ever-increasing volume and diversity of data will inevitably lead to a non-linear increase in management complexity and performance requirements. An AI data lake solution running on a three-layer architecture can effortlessly address these challenges to facilitate the development of large AI models, accelerate the emergence of intelligence in model training, and push AI innovation and development to new heights.
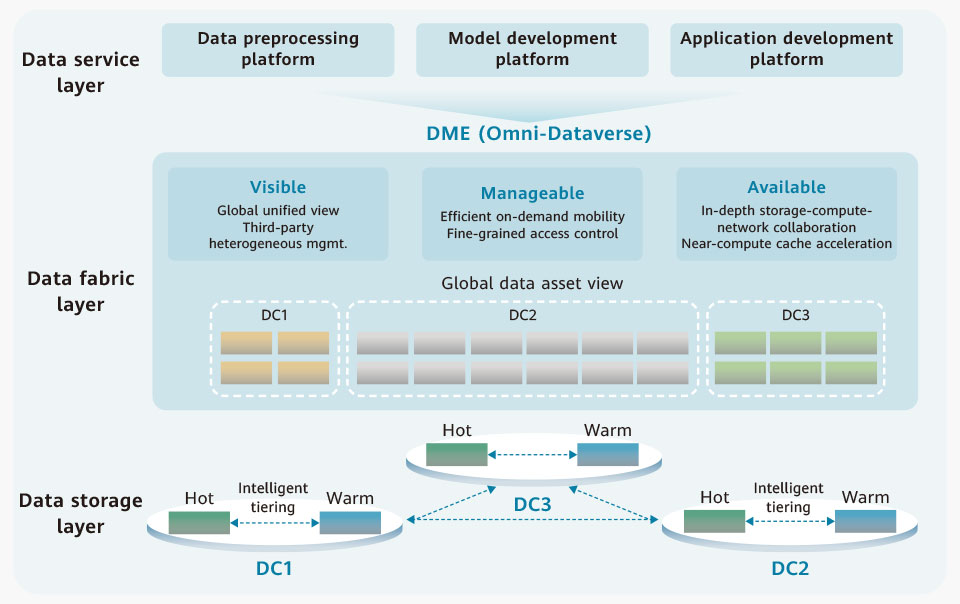
Figure 3: Architecture of Huawei AI data lake solution
- Tags:
- AI
- Data Storage