Industry Trends
AI-ready Cloud Drives Intelligent Digital Transformation for Carriers
To achieve business success through digital and intelligent transformation, carriers need to be AI-ready in terms of corporate architecture, business models, and infrastructure.

By Wang Xiaobin, Chief Architect, ICT Computing Products and Solutions (Carrier Domain), Huawei
ChatGPT: Igniting AI models globally
ChatGPT provides a complex mix of answers that can be amazing, amusing, or utterly wrong.
GPTs have put AI, an industry with a history of over 70 years, under the spotlight again. The emergence of ChatGPT is regarded as a turning point for AI, transitioning it from a technology that understands the world to one that shapes it. The integration of technologies, such as scaling laws, reinforcement learning, and human feedback, has given ChatGPT human-like inference capabilities.
OpenAI went on to launch a series of innovative products, including GPT-4 (1.8 trillion parameters), GPT-4 Turbo, Assistants API, ChatGPT Enterprise, and GPTs for ChatGPT in 2023, as well as Project Q* and Sora, which were just disclosed. Other players on the industry value chain are also accelerating innovation: Humane launched AI Pin, Microsoft released the generative AI office suite Microsoft 365 Copilot, and Google DeepMind launched Gemini. These are accelerating the application of foundation models to industry and individual markets and guiding AI development towards multimodality and intelligent agents.
In China, many major tech companies have joined the AI race by launching their own foundation models. These include Baidu's Ernie Bot, Alibaba's Tongyi Qianwen, Huawei's Pangu, and 360's Smart Brain, Kunlun Tech's Skywork, JD's ChatRhino, iFLYTEK's Spark, Tencent's Hunyuan, and SenseTime's SenseNova. In just six months, the number of foundation models in China increased from around 100 to more than 200. Many SMEs have also released vertical models, including Trip.com's Xiecheng Wendao for tourism, NetEase's Ziyue for education, JD Health's Jingyi Qianxun for healthcare, and Ant Group's finance AI model. These are facilitating the evolution of AI capabilities from perception to cognition and from identification to generation, while expanding the scope of AI applications from general-purpose to industry-specific. AI is expected to be applied to over half of core industry scenarios over the next two years.
We can already see the potential of AI for reshaping all services in the carrier industry. Since the marginal cost of foundation models approaches 0, applying AI to internal operations can help carriers reduce costs and improve efficiency. Leveraging AI in B2C, B2H, and B2B services will allow carriers to generate higher revenue. For example, AI-powered CCTV solutions can create a 15%-plus premium over traditional solutions, and the AI-powered 5G New Calling service can generate more than 10% higher ARPU. AI is accelerating carrier digital transformation with carriers around the world evolving cloud-based transformation to cloud and AI-based intelligent digital transformation.
Lower costs, better efficiency, and higher revenue
To seize the enormous opportunities presented by AI, carriers require advancements in three areas to be AI-ready.
Making AI part of the transformation strategy and creating an AI-ready technology architecture
Carriers must make AI a key part of their digital transformation and overall transformation strategy, with specialized teams in place to implement AI strategies and build AI-oriented enterprise architectures and capabilities. TOGAF is an enterprise architecture framework that bridges enterprise strategic planning and IT solutions, and serves as the core of enterprise digitalization. This framework consists of four components: business architecture (BA), information/data architecture (IA), application architecture (AA), and technology architecture (TA). Without an enterprise architecture that maps strategic objectives to execution, digital transformation is unlikely to succeed. Therefore, it is recommended that carriers integrate AI into their enterprise architecture so that AI can power their strategies, businesses, data, and technologies.
At the same time, the major initiative of digital transformation may encounter large setbacks during execution. Involvement and promotion by top executives will be key to making carriers AI-ready. Huawei, for example, announced its AI strategy at HUAWEI CONNECT in 2018. In that same year, the company's founder Ren Zhengfei issued a resolution on increasing investment in AI and using AI to improve corporate efficiency, establishing the AI Enabling Dept headed by Ren himself. Today, Huawei has already made AI part of every business process in every department and business unit. To date, Huawei has used AI to create more than 600 intelligent applications in over 80 business settings, build nearly 7,000 AI models, and create over 20,000 digital employees that serve Huawei organizations in more than 170 countries and regions around the world. Huawei significantly reduces operation costs with a company-wide foundation model-based intelligent agent that gives all employees access to an AI assistant.
In terms of products, the AITO M9, which is equipped with Huawei's intelligent driving solution, caused a sensation in the new energy vehicle market in 2023. The voice assistant Celia, which is pre-installed in every Huawei smartphone, improves user experience. AI-enabled energy-saving algorithms allow Huawei's wireless base stations to consume over 20% less energy than competing products, in turn driving up carriers' revenues. In the carrier industry, China Telecom and China Mobile also made "AI+" part of their group strategy in 2023.
Using platforms to create AI-ready business models, promote industry collaboration, and foster a thriving ecosystem
There is no lack of companies or other players who are actively exploring applications for foundation models. Explorations into new AI applications for industries will likely result in high trial-and-error costs and even failure. Although carriers can participate in creating foundation models, their greater strength lies in ubiquitous cloud-network infrastructure that can serve as supporting and monetization platforms for foundation models, as well as existing industry customers that can form a potential market as carriers look to commercialize foundation models. Carriers' computing and O&M capabilities and credibility in local communities give them the potential to provide professional platforms for local AI markets. With such platform capabilities, carriers can engage and aggregate foundation model providers for business exploration and provide the Model as a Service (MaaS) business model (Figure 1). This will enable monetization, encourage more partners to sell their model products on the platform to form a long tail market,prevent carriers from having to make foundation models themselves that compete with their partners, and reduce trial-and-error costs for uncertain models.
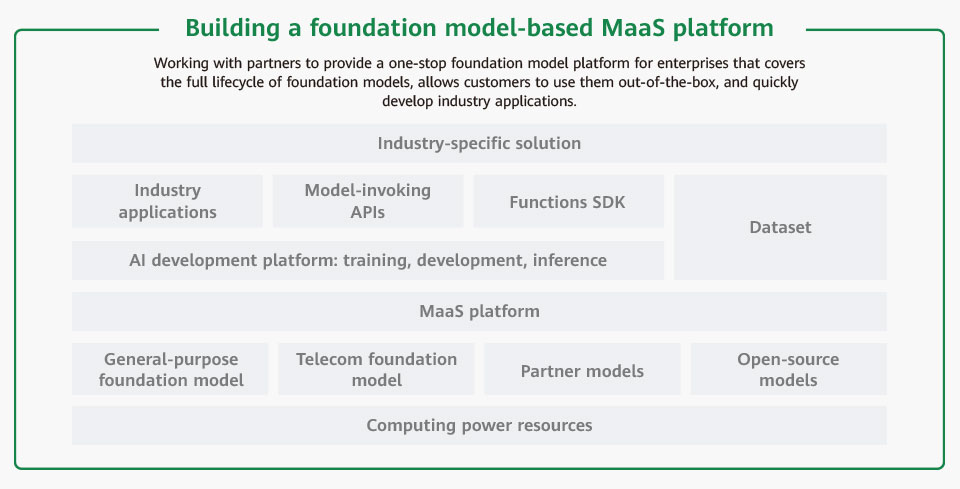
Figure 1: Foundation model-based MaaS platform capability framework
China Telecom, for example, launched a foundation model ecosystem cooperation alliance in July 2023 to help implement China's cloudification and digital transformation strategy and accelerate the construction of ICT infrastructure centered on general computing, AI computing, and supercomputing. This alliance publicly engaged influential foundation model partners across the industry. At the Digital Technology Ecosystem Conference in November 2023, China Telecom and its partners launched the first batch of 12 industry-specific foundation models for trial commercial use, which involved sectors like education, construction, finance, and mining. These foundation models are all embedded on the Xingchen MaaS ecosystem service platform. By integrating networks, cloud, intelligent computing, AI, and partner models, this business model is driving the intelligent upgrade of Telecom China's cloud services and is already generating commercial returns.
For individual model developers, carriers should consider taking the lead to develop a foundation model ecosystem community similar to Hugging Face to maximize the ecosystem value of foundation models across society. Reasonable industrial division, platform building, and ecosystem development should be key objectives of carriers in the AI foundation model era.
Building AI-ready infrastructure with AI-powered clouds and data
In the future, foundation models will provide ubiquitous intelligent services through tremendously diverse business models. These foundation models can be embedded in a variety of software and hardware systems, such as intelligent vehicles and robots, to make their way into commercial markets in the form of intelligent products. They can also be deployed in the cloud to provide cloud services based on commercial foundation models and transform existing business logic. Cloud and network infrastructure are the pipes through which AI can reach end users (consumers, businesses, and homes). AI can only reach where cloud and networks are available, so an AI-ready intelligent cloud is needed for carriers to enter the AI industry. For carriers that are engaged in the AI business, cloud will be essential for AI in the early stages, but AI will become a key part of cloud further down the road. Over the past few years, China Telecom has created a nationwide e-Cloud service. In March 2024, China Telecom's Chairman Ke Ruiwen said at the company's 2023 Annual Results Announcement, "Without AI, cloud does not have a future." He also claimed that e-Cloud will accelerate the upgrade to intelligent cloud to become a leading foundation model computing service provider in China.
Carriers should build AI-ready intelligent cloud solutions based on the three key elements of AI: computing power, data, and algorithms. An AI-ready cloud platform should feature one architecture and two capabilities:
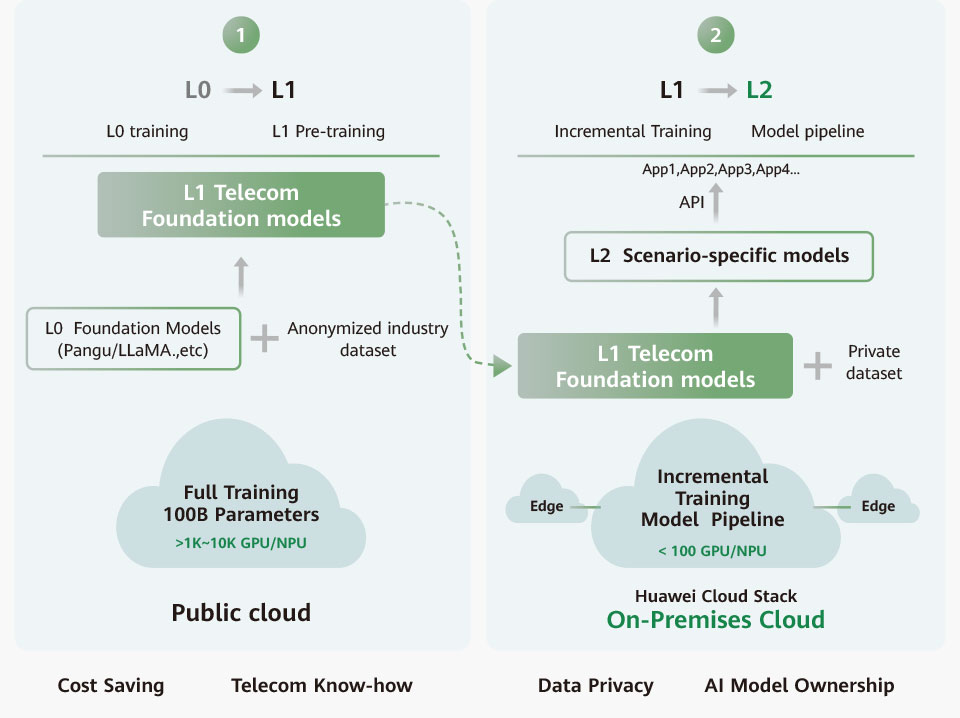
Figure 2: Customized models based on collaborative training on public and private clouds
-
Computing power: Distributed multi-layer cloud architecture for ubiquitous computing power
Distributed multi-layer cloud architecture requires collaboration between the public cloud, on-premises cloud, and edge cloud. Full training is performed on the public cloud, incremental training and centralized inference on the on-premises cloud, and other inference on the edge clouds (Figure 2). This architecture brings three benefits for carriers to deploy foundation models.
- Reduced costs: The pre-training of foundation models requires huge amounts of AI computing power (thousands of GPUs) within a certain period of time (weeks or months). For example, the LLaMA 2 70B model was trained using a distributed supercomputing cluster with 2,000 NVIDIA A100 GPUs, while the Falcon 180B model used 4,096 NVIDIA A100 GPUs. Most carriers, especially those outside China, do not need to purchase computing power in advance for this purpose. They can instead lease public cloud computing power. This allows them to perform full training with the 10,000-GPU computing power already deployed on the public cloud and publicly available industry datasets. Pre-trained foundation models can be deployed on the on-premises cloud where incremental training can be performed based on a small amount of private data of carriers or specific industries. This exponentially reduces the required hardware investment (dozens of GPUs). This model featuring full training on the public cloud and incremental training on on-premises clouds means a 100-fold saving on investment in computing power.
- More secure data: Laws and regulations in many countries prohibit data (such as network information and traffic information), which usually is high-quality datasets for model training, from being transferred out of the local network or abroad. On-premises clouds and edge clouds can meet these requirements. Foundation models trained on such clouds are already built on privacy and sensitive data alongside inherent knowledge. This means they run locally and privately, ensuring data security.
- Better service experience: Edge clouds can bring AI inference closer to end users and provide lower-latency experiences.
- Reduced costs: The pre-training of foundation models requires huge amounts of AI computing power (thousands of GPUs) within a certain period of time (weeks or months). For example, the LLaMA 2 70B model was trained using a distributed supercomputing cluster with 2,000 NVIDIA A100 GPUs, while the Falcon 180B model used 4,096 NVIDIA A100 GPUs. Most carriers, especially those outside China, do not need to purchase computing power in advance for this purpose. They can instead lease public cloud computing power. This allows them to perform full training with the 10,000-GPU computing power already deployed on the public cloud and publicly available industry datasets. Pre-trained foundation models can be deployed on the on-premises cloud where incremental training can be performed based on a small amount of private data of carriers or specific industries. This exponentially reduces the required hardware investment (dozens of GPUs). This model featuring full training on the public cloud and incremental training on on-premises clouds means a 100-fold saving on investment in computing power.
Data: Cloud-based data production line for efficient data processing
While data is a core asset of carriers, it is scattered in independent IT systems and does not flow. Not only are there hardware costs for storing data, its value remains untapped. Local cloud services require full-lifecycle one-stop data governance capabilities (Figure 3), including pool-based data storage and cross-domain collaborative data scheduling and management. Data lake-warehouse convergence can help create a logical data lake that brings together scattered data lakes and warehouses. This makes it possible for one copy of data to be shared by multiple data analytics engines and AI engines. It also enables the development of model training-oriented data processing capabilities, such as the efficient integration, cleaning, filtering, and labeling of datasets.
Algorithms: Cloud-based model production ine enabling lifecycle management of foundation models
The lifecycle of a foundation model includes key phases such as development, training, fine-tuning, and deployment. Tools and capabilities adapted to these phases should be deployed on the on-premises cloud as services. Simply put, the on-premises cloud should enable the development, training, fine-tuning, and deployment of foundation models as services to help carriers manage models (Figure 4). When it comes to ecosystem compatibility, cloud services should support major open-source models. Carriers need to build independent model-related capabilities that can ensure data security and the manageability and controllability of models.
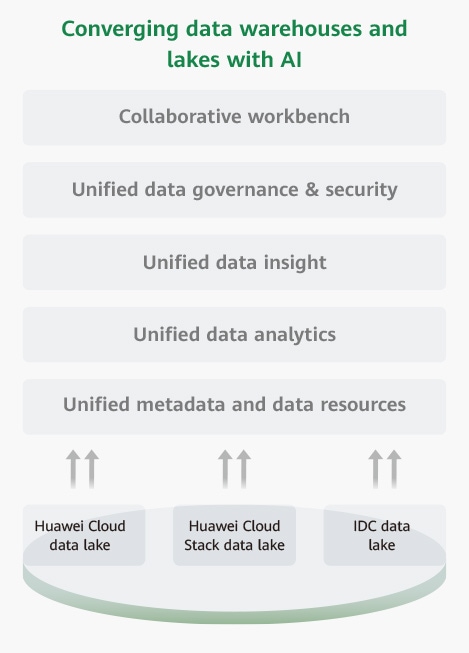
Figure 3: Data-AI convergence: An efficient cloud-based
data foundation for the AI era
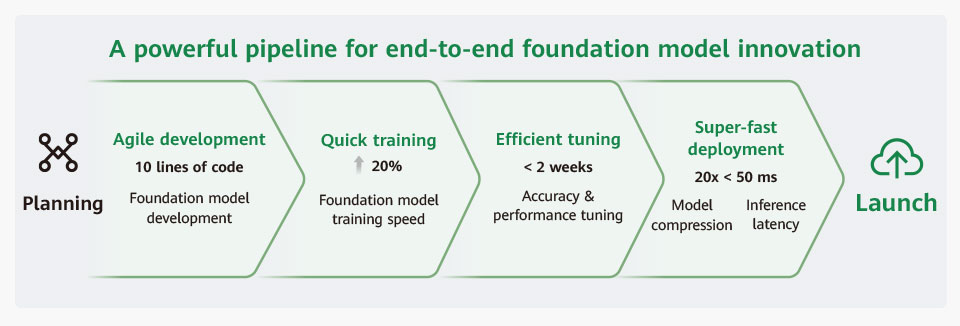
Figure 4: A powerful pipeline for end-to-end foundation model innovation
We are stepping faster into an AI era as the fourth industrial revolution approaches. Carriers need to be AI-ready in terms of strategy, organization, capability, and infrastructure. They should use their cloud-network strengths, position themselves appropriately in the industrial division of work, and build their ecosystem platform capabilities to provide Model as a Service. This will allow them to bring together more industry partners and succeed in intelligent digital transformation.