Methodology
Humankind has always been driven to explore, and bring certainty to this uncertain world. We believe that collaboration is essential for future exploration. Over the past three years, in addition to our own Huawei experts who have been building their own insights and plans for the future, our GIV@2030 research team has also been communicating extensively with industry scholars, customers, and partners. We have studied data, methodologies, and insight reports from prominent players around the world, including the United Nations, GSMA, and other third-party consulting firms. The GIV@2030 research team used trend extrapolation and time series forecasting to arrive at their findings about the future.

Huawei GIV Predictions for 2030 – L1
No. | Indicator | Definition | Prediction for 2030 |
---|---|---|---|
1 | Number of global connections | Total number of connected people and things worldwide | 200 billion |
2 | Number of wireless cellular connections | Total number of people and things connected through wireless cellular technologies worldwide | 32.5 billion cellular connections and 80 billion cellular-based passive connections |
3 | Penetration rate of gigabit/10 gigabit home broadband | Proportion of gigabit or higher home broadband users to global households, and proportion of 10 gigabit home broadband users to global households | Penetration rate of gigabit or higher home broadband: 60%; penetration rate of 10 gigabit home broadband: 25% |
4 | Number of active wireless AI agent users worldwide | Number of network users with AI agents | 6 billion |
5 | IPv6 address penetration rate | Percentage of connections and applications using IPv6 addresses worldwide | 90% |
6 | Number of VR/AR/MR users | Total number of VR/AR users worldwide | 1 billion |
7 | Number of robots per 10,000 workers working together | Number of robots per 10,000 manufacturing workers working together | 1000 |
8 | C-V2X penetration rate | Proportion of C-V2X vehicles to vehicle parc | 60% |
9 | Monthly wireless cellular network traffic per user | Average monthly cellular network traffic per user (GB), including users using multiple devices | 600 GB, 40x more than 2020 |
10 | Monthly average home network traffic | Average monthly network usage of a family | 1.3 TB, 8x more than 2020 |
11 | Number and penetration rate of 5G industry private networks | Number of 5G industry private networks (including virtual private networks)/Proportion of large- and medium-sized enterprises using 5G industry private networks | Number of 5G industry private networks: 1 million; penetration rate of 5G industry private networks among large- and medium-sized enterprises: 35% |
12 | Penetration rate of gigabit/10 gigabit enterprise Wi-Fi | Proportion of 1 Gbps and 10 Gbps bandwidths for WLAN access on enterprise campuses | Gigabit enterprise Wi-Fi penetration rate: 14%; 10 gigabit enterprise Wi-Fi penetration rate: 84% |
13 | FTTR broadband penetration rate | Number of global FTTR-H home users Number of global SME FTTR-B users |
FTTR-H home broadband penetration rate: 31% FTTR-B broadband penetration rate of SMEs: 41% |
14 | Average AN level of carriers | Average AN level of global carriers | L4 |
15 | Global penetration rate of home cloud storage | Percentage of high-speed cloud storage in home scenarios | 35% |
16 | Penetration rate of home cloud computer services | Percentage of cloud computers in home scenarios | 17% |
17 | Home security service penetration rate | 1. Percentage of households with cameras installed 2. Percentage of households with 3D optical sensing terminals installed |
1. 2030: China: 24%; global: 15% 2. 2030: 3D optical sensing (elderly care) penetration rate: 8% |
18 | Number of global fiber broadband users | Number of global fiber broadband users | 1.6 billion |
19 | OTN coverage rate of transmission networks in large enterprises, public institutions, and government agencies | OTN coverage rate of transmission networks in key universities and scientific research institutions, large hospitals, and large industrial enterprises, as well as development zones and industrial parks at the county level or above. | 100% |
20 | Density of OTN integrated access nodes per 10,000 people | Number of OTN integrated access nodes per 10,000 people | Number of integrated access nodes per 10,000 people: 2 by 2025 and 4 by 2030 |
21 | Density of 100G OTN integrated access nodes per 10,000 people | Number of 100G OTN integrated access nodes per 10,000 people | 1 |
22 | Reliability of network connections for distributed AI foundation model training | Reliability of DCI connections required for distributed training between different data centers | 99.9999% |
No. | Indicator | Definition | Prediction for 2030 |
---|---|---|---|
23 | Proportion of unstructured data used for decision-making in production | The proportion of unstructured data (text, images, videos, and audio) used for decision-making in production | 80% |
24 | Proportion of enterprises deploying multi-layer ransomware protection systems covering the storage systems | The proportion of enterprises that deploy multi-layer ransomware protection systems | >80% |
25 | Proportion of hot data stored on SSDs | The proportion of hot data stored on SSDs after usage | 100% |
26 | Proportion of enterprises accessing active archived data at least once a day | The proportion of enterprises that access active archived data at least once a day | >60% |
27 | Proportion of the cloud and Internet enterprises using diskless reference architectures | The proportion of the cloud and Internet enterprises that adopt diskless reference architectures for IT systems | >80% |
28 | Proportion of endpoint, edge, and core data center data processed by AI in real time | The proportion of endpoint, edge, and core data center data that is processed by AI in real time | >75%,>80%,>90% |
No. | Indicator | Definition | Prediction for 2030 |
---|---|---|---|
29 | Cloud resource utilization enhanced by flexible compute | Cloud resource utilization enhanced by flexible compute | 70% |
30 | Peer-to-peer architecture penetration in cloud data centers | Proportion of cloud data centers that evolve from primary/secondary architecture to peer-to-peer architecture | 60% |
31 | Green power penetration in cloud data centers | Proportion of cloud data centers that use new technologies such as liquid cooling, thermal storage, waste heat recovery, and energy storage, as well as renewable energy such as solar, wind, and nuclear energy | 100% |
32 | AI devices facilitating device-cloud synergy | Number of devices that offer advanced intelligent assistance powered by the robust cloud computing power and electric power, such as intelligent connected vehicles | 3 billion |
33 | "Cloud for devices" compute capacity | Total compute capacity offered by cloud data centers to devices | 40ZFlops |
34 | Contribution of AI to the GDP from creative and knowledge-intensive industries | Contribution of AI to the GDP from creative and knowledge-intensive industries | 75% |
35 | AI penetration in scientific computing tasks | AI penetration in scientific computing tasks | 50% |
36 | Discriminative AI penetration in all AI use cases | Discriminative AI penetration in all AI use cases | 50% |
37 | Discriminative AI penetration in all enterprise use cases | Discriminative AI penetration in all enterprise use cases | 70% |
38 | Media content AI-generated or generated with the support of AI | Media content generated by AI or with the support of AI | 70% |
39 | Jobs impacted by AI agents | Jobs in the world impacted by AI agents | 67% |
40 | Work hours replaced by AI agents | Work hours that AI agents may replace in total economic working hours | 30% |
41 | People who need to be reskilled for jobs due to AI | People who need to be reskilled for jobs under the impact of AI | > 40 million |
42 | Employees who will have their own intelligent assistants | Employees who will have their own intelligent assistants | 1.5 billion |
43 | XR device shipment | XR devices shipped globally | 60 million |
44 | People who venture into the realm of spatial computing, a fusion of virtual and real worlds | People across the globe who venture into the realm of spatial computing, a fusion of virtual and real worlds | 500 million |
45 | Additional hours spent on spatial intelligence | Average additional hours of each person spent on spatial intelligence everyday | 5 hours/day |
46 | Areas covered by city-level 3D reconstruction solutions | Areas covered by city-level 3D reconstruction solutions with AI+CG | 10,000 square kilometers |
47 | Compute demand from data preprocessing and training of VLA models | Compute demand from data preprocessing and training of VLA models that power spatial intelligence | 100Z Flops |
48 | General-purpose service robot shipment | Shipment volume of general-purpose service robots powered by embodied AI | 30 to 50 million units |
49 | Enterprise software built or rebuilt with AI | Enterprise software built or rebuilt with AI | 80% |
50 | Global digital content producers assisted by AI + CG | Global digital content producers assisted by AI + CG | 100 million |
51 | Interactive media content created with AI + CG | Length of interactive media content created with AI + CG | 1 trillion hours |
52 | Software development efficiency increase with convergence pipelines | Efficiency increase of different software development after large-scale application of convergence pipelines | 10-100 times |
53 | Man-machine speech recognition accuracy | Man-machine speech recognition accuracy enabled with natural language processing (NLP) | >98% |
54 | Market space of smart contract platforms | Market space of smart contract platforms that execute and manage an array of functions and services | USD15–25 trillion |
55 | Web 3.0 zero-knowledge proofs | Zero-knowledge proofs executed by Web 3.0 applications | > 90 billion |
56 | Smooth cloud migration efficiency increase for applications | Efficiency increase due to fully automated and unattended cloud migration for applications | 10 times |
57 | Cloud governance compliance risks eliminated in the early stages | Cloud governance compliance risks eliminated in the early stages using AI and other technologies | 90% |
58 | Faults that can be detected within 1 minute, demarcated within 5, and recovered within 10 | Faults that can be detected within 1 minute, demarcated within 5, and recovered within 10 | 80% |
59 | Cloud resource expenses saved with FinOps | Cloud resource expenses saved for global users every year with FinOps | > USD200 billion |
60 | Increase in intensity and complexity of cyber attacks | Increase in intensity and complexity of cyber attacks across the globe | 10 times |
61 | Companies that will implement zero trust policies with cloud security as the core | Global companies that will implement zero trust policies with cloud security as the core | >95% |
62 | Organizations that will adopt AI-powered cybersecurity products | Global organizations that will adopt AI-powered cybersecurity products | 80% |
63 | Companies that have their own security models | Global companies that have their own security models | 50% |
64 | Companies that adopt an AI-powered cloud native SecOps model | Global companies that adopt an AI-powered cloud native SecOps model | 70% |
No. | Indicator | Definition | Prediction for 2030 |
---|---|---|---|
65 | Proportion of renewables in global electricity generation | The proportion of electricity from renewables in the total electricity generated worldwide | 65% |
66 | Global total PV installations | The total capacity of PV plants installed worldwide | 6000GW |
67 | Proportion of digital infrastructure using green energy | The proportion of digital infrastructure that is powered by green energy | >80% |
68 | Proportion of PV plants using AI technologies | The proportion of PV plants that deploy AI technologies | 90% |
69 | Number of ultra-fast charging vehicle models | The number of vehicles that support ultra-fast charging | >60% |
70 | Annual ESS capacity addition | The total capacity of energy storage systems added each year worldwide | 140GW |
71 | Annual energy charged to EVs | The total amount of energy charged to electric vehicles each year | 1.1 trillion kWh |
No. | Indicator | Definition | Prediction for 2030 |
---|---|---|---|
72 | Autonomous vehicles as a proportion of new vehicles sold | Autonomous vehicles as a proportion of new vehicles sold annually in China | L2: 90%; L3 and higher: 30% |
72 | Electric vehicles as a porportion of new vehicles sold | Electric vehicles as a porportion of new vehicles sold annually in China | 82% |
74 | In-vehicle computing power | Computing capabilities within a vehicle | Over 5000 TOPS |
75 | General obstacle detection accuracy | Accuracy of detecting general obstacles during intelligent driving | >99% |
76 | Safety of intelligent driving surpassing human driving | Level of safety of intelligent driving compared to human driving (measured by accidents) | 10 times safer than human driving |
77 | Miles per intervention | How far a vehicle can travel before human intervention is required during the intelligent driving process | 621 miles (equivalent to 1000 km) |
78 | Daily driving distance simulated in system | Daily virtual simulation capability of the model for simulating interactions between traffic participants constructed in the simulation system | Over 6.21 billion miles (equivalent to 10 billion km) |
79 | Daily driving distance achieved by cloud-based parallel simulation | Intelligent driving testing and verification capability built in the cloud | Over 6.21 million miles (equivalent to 10 million km) |
80 | Improvement of E2E response delay | Comparison of the end-to-end response delay capability of intelligent driving and human driving | Two times improved |
No. | Indicator | Definition | Prediction for 2030 |
---|---|---|---|
81 | Proportion of AI computing power | Proportion of AI computing power to final power in the power system | 90% |
82 | Edge intelligence adoption | Intelligent edge deployment rate in the power system | >75% |
83 | Proportion of cloud-based applications of electric power companies | Degree of cloud-based resources, platforms, and applications in power systems | >75% |
84 | Coverage of next-generation HPLC technology | Application ratio of next-generation HPLC technology in power systems | 100% |
No. | Indicator | Definition | Prediction for 2030 |
---|---|---|---|
85 | O&M agent coverage | Proportion of carrier ICT O&M scenarios with agents | 45% |
86 | O&M copilot coverage | Proportion of carrier ICT O&M scenarios with copilots | 100% |
87 | Digital twin penetration rate | Proportion of carriers that have deployed digital twin systems | 30% |
No. | Indicator | Definition | Prediction for 2030 |
---|---|---|---|
88 | General-purpose computing power of a cluster | Effective computing power of a single cluster with software and hardware tuning | >70EFplops |
89 | AI computing power of a cluster | Effective computing power of a single AI cluster | 750EFlops |
90 | Cluster storage capacity | Effective storage capacity of a single cluster | Exabytes |
91 | Percentage of data collaboratively processed by clouds and edges | Ratio of data requiring edge and data center processing to the total data volume | 80% |
92 | Digital access rate of enterprises' production devices | Ratio of enterprises' production devices that can be accessed through edge data centers to enterprises' total production devices, after being IoT-enabled and intelligentized | 80% |
93 | Data security investment ratio | Proportion of data security investment to the total data center investment | 20% |
94 | System-level availability | System-level availability = System's annual MTBF/(System's annual MTBF + MTTR)(MTBF is short for mean time between failures, and MTTR is short for mean time to repair) | 99.999% |
95 | Disaster recovery (DR) coverage of important data | Percentage of important data and associated application systems for which DR is available | 100% |
96 | Automation level | L1: human assisted; L2: partially automated; L3: conditionally automated; L4: highly automated; L5: fully automated. (Automation includes automatic predictive troubleshooting and analysis, automatic emergency handling, and AI-powered energy efficiency management. L4 indicates operations approaching truly unmanned, and L5 indicates operations without any human involvement.) | L4 |
97 | Power usage effectiveness (PUE) | Total data center power consumption/IT equipment power consumption | 1.0x |
98 | Renewable energy factor (REF) | Renewable energy consumption/Total data center power consumption | 80% |
99 | Water usage effectiveness (WUE) | Water consumption/IT equipment power consumption | 0.5 L/kWh |
100 | DC-level resource pooling rate | Ratio of compute, storage, and network resources available for global scheduling to all resources in a single DC | 80% |
101 | Proportion of new cloudnative applications | Ratio of new cloud-native applications to all new applications | 90% |
102 | Resource allocation granularity | Granularity of compute, storage, and network resource allocation, scheduling, and billing | Functionlevel |
103 | Penetration rate of hyper-converged interconnection bus technologies | Penetration rate of unified hyper-converged interconnection bus technologies | 60% |
104 | Penetration rate of hyper-converged Ethernet networks | Ratio of converged networks of general-purpose computing, high-performance computing, and storage to all networks of data centers | 80% |
105 | Penetration rate of optical + computing collaboration | Ratio of cluster computing power that supports computing power collaboration using spine layer on the all-optical direct connection AI parameter plane to the total computing power of the cluster | 50% |
106 | Penetration rate of optical + storage collaboration | Ratio of data transmitted in cross-WAN highspeed mode using all-optical direct connection SSD to the total transmitted data | 50% |
107 | Percentage of all-flash storage | Ratio of all-flash storage capacity to the total capacity of data centers | 80% |
108 | Penetration rate of RDMA storage networks | Percentage of RDMA-based storage networks | 80% |
109 | Percentage of data processed near memory or in memory | Ratio of the amount of data processed near memory or in memory to the total amount of data processed | 30% |
No. | Indicator | Definition | Prediction for 2030 |
---|---|---|---|
110 | Intelligent platform utilization | Percentage of campuses in China that use intelligent platforms | 30% |
111 | Light field holographic rendering computing power | A single user's demand for light field holographic rendering computing power in digital twins | 10TFLOPS |
112 | AI penetration rate | AI penetration rate of new campus services | 100% |
113 | Optically-sensed gas density precision | Gas density sensing precision of the HCS system that combines communications with IoT sensors | <10ppm |
114 | Optically-sensed illuminance precision | Illuminance sensing precision of the HCS system that combines communications with IoT sensors | <0.1lux |
115 | Optically-sensed humidity precision | Humidity sensing precision of the HCS system that combines communications with IoT sensors | <2%rh |
116 | Optically-sensed temperature precision | Temperature sensing precision of the HCS system that combines communications with IoT sensors | <0.2°C |
117 | Human body imaging precision of radar | Precision of detecting human body position and contour based on multi-radar collaboration | Centimeterlevel imaging |
118 | Number of XR users | User quantity of XR, which is an important means to support immersive interaction | 1 billion |
119 | TSN jitters and latency | Necessary jitters and latency from sensing to collaboration of human-machine interaction supported by asynchronous TSN | 10us-100us |
120 | Miniature holographic bandwidth | Bandwidth capacity required to display a 10-inch miniature hologram | 12.6G |
121 | Simulation computing power | Single-user computing power required to shorten network latency through computing to simulate real user behaviors | 1 TeraFLOPS |
122 | Area of the map drawn in real time | The capability of the data foundation service to draw a spatial map in real time | 1 million square meters |
123 | Agent density of real-time interaction | Number of agents that support real-time interaction in the same physical space | 10000 |
124 | Wireless access bandwidth on campuses | Peak rate of an AP and cell capacity of the mobile network | 100 Gbit/s |
125 | Deterministic latency | Wireless air interface latency of an AP 1 ms with | 1 ms with reliability of 99.9999% |
126 | Multi-modal wireless coverage | The medium coverage distance of multi-modal Wi-Fi with microwatt-level power consumption | 2-10km |
127 | 10GE Wi-Fi penetration rate | Global utilization of 10GE Wi-Fi on enterprise campuses | 84% |
128 | Penetration rate of 5G industry private network | Global utilization of 5G industry private network on enterprise campuses | 35% |
129 | Proportion of investment in data security | Proportion of investment in data security | 20% |
130 | Campus PV LCOE | LCOE of PV power generation on campuses | US$0.01 per kWh |
CGE Model
Computable general equilibrium (CGE) model is an economic modelling framework that incorporates both the demand and supply-side factors and uses actual economic data to estimate how an economy might react to changes in policy, technology or other external factors.
On the supply-side the CGE model allows us to model the economic impacts of productivity enhancements generated by ICT as a result is well-suited to estimate the economic impacts of ICT and and take into account the interactions and knock-on effects between its different industries.
The CGE model does include carbon accounting, as such we can demonstrate the contribution ICT adoption will have on carbon emissions and the relative carbon intensity of industry.
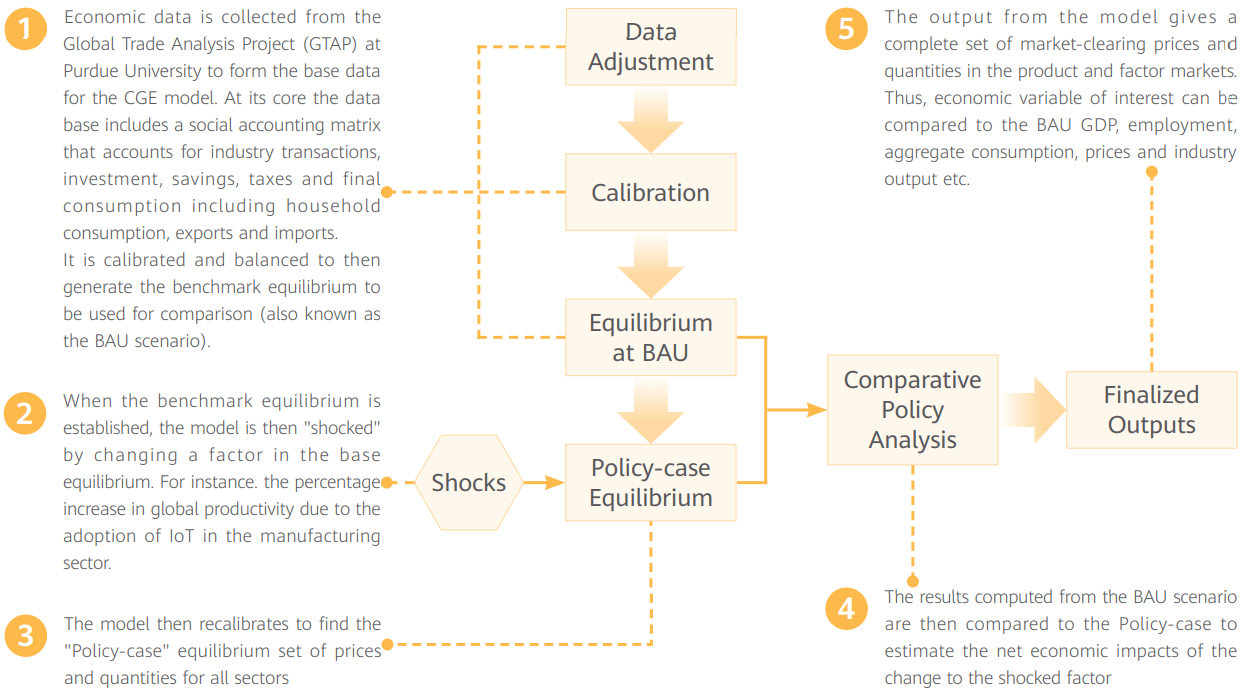